Keratoconus Disease Prediction by Utilizing Feature-Based Recurrent Neural Network
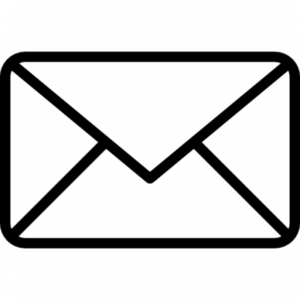
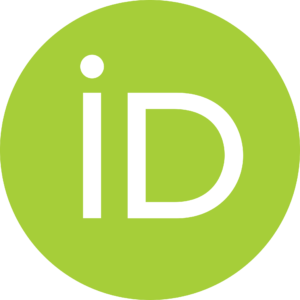
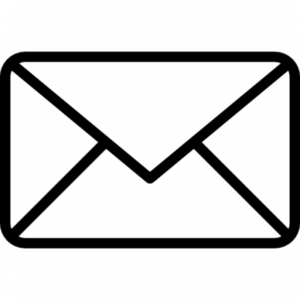
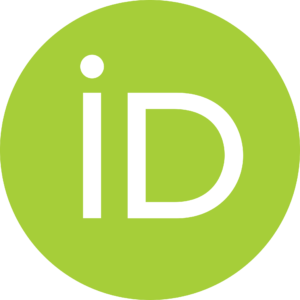
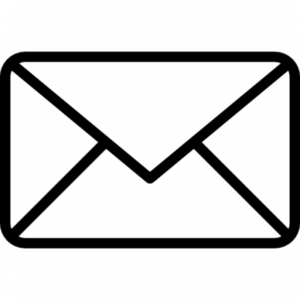
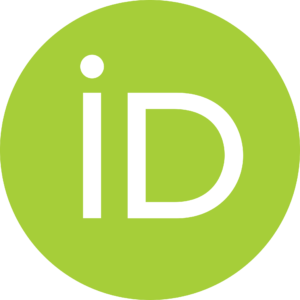
1 Department of Electrical Engineering University of Islamic Azad University, Isfahan (Khorasgan) Branch, Isfahan, Iran
2 Department of Electrical and Electronic Engineering Faculty of Istanbul Aydin University, Istanbul, Turkey
* Author to whom correspondence should be addressed.
Journal of Engineering Research and Sciences, Volume 3, Issue 7, Page # 44-52, 2024; DOI: 10.55708/js0307004 Keywords: Cornea disease, Feature extraction, Keratoconus prediction Received: 30 April, 2024, Revised: 04 July, 2024, Accepted: 05 July, 2024, Published Online: 19 July, 2024APA Style
Musa, S. H., Jaafar, Q., Alhaidar, M., Mahdi, M., & Elmi, B. (2024). Keratoconus disease prediction by utilizing feature-based recurrent neural network. Journal of Engineering Research and Sciences, 3(7), 44-52. https://doi.org/10.55708/js0307004
Chicago/Turabian Style
Musa, Saja Hassan, Qaderiya Jaafar, Mohammed Alhaidar, Mohammad Mahdi, and Borhan Elmi. “Keratoconus Disease Prediction by Utilizing Feature-Based Recurrent Neural Network.” Journal of Engineering Research and Sciences 3, no. 7 (2024): 44-52. https://doi.org/10.55708/js0307004.
IEEE Style
S. H. Musa, Q. Jaafar, M. Alhaidar, M. Mahdi, and B. Elmi, “Keratoconus Disease Prediction by Utilizing Feature-Based Recurrent Neural Network,” Journal of Engineering Research and Sciences, vol. 3, no. 7, pp. 44-52, 2024, doi: 10.55708/js0307004.
Keratoconus is a noninflammatory disorder marked by gradual corneal thinning, distortion, and scarring. Vision is significantly distorted in advanced case, so an accurate diagnosis in early stages has a great importance and avoid complications after the refractive surgery. In this project, a novel approach for detecting Keratoconus from clinical images was presented. In this regard, 900 images of Cornea were used and seven morphological features consist of area, majoraxislength, minoraxislength, convexarea, perimeter, eccentricity and extent are defined. For reducing the high dimensionality datasets without deteriorate the information significantly, principal component analysis (PCA) as a powerful tool was used and the contribution of different PCs are determined. In this regard, Box plot, Covariance matrix, Pair plot, Scree Plot and Pareto plot were used for realizing the relation between different features. Improved recurrent neural network (RNN) with Grey Wolf optimization method was used for classification. Based on the obtained results, the average prediction error of the visual characteristics of a patient with keratoconus six and twelve months after the Kraring ring implantation using RNN are 9.82% and 9.29%, respectively. The average error of estimating characteristics of predicting the visual characteristics of a patient with keratoconus six and twelve months after myoring ring implantation are 11.46% and 7.47% respectively.
- J. Santodomingo-Rubido, G. Carracedo, A. Suzaki, C. Villa-Collar, S. J. Vincent, “Keratoconus: An updated review,” Contact Lens and Anterior Eye, vol. 45, no. 3, 101559, 2022, doi.org/10.1016/j.clae.2021.101559.
- A. Lavric, and P. Valentin, “KeratoDetect: keratoconus detection algorithm using convolutional neural networks.”, Computational intelligence and neuroscience, 2019, doi.org/10.1155/2019/8162567.
- M. M. Vandevenne et al., “Artificial intelligence for detecting keratoconus”, Cochrane Database of Systematic Reviews 11, 2023, doi.org/10.1002/14651858.CD014911.pub2.
- V. Galvis, T. Sherwin, A. Tello, J. Merayo, R. Barrera, and A. Acera, “Keratoconus: an inflammatory disorder?.” Eye 29, no.7, 2015, 843-859, doi.org/10.1038/eye.2015.63.
- M.J. Kaisania, “A machine learning approach for keratoconus detection.” (Ph. D Thesis, 2021).
- P. J. Shih, H. J. Shih, I. J. Wang, and S. W. Chang. “The extraction and application of antisymmetric characteristics of the cornea during air-puff perturbations,” Computers in Biology and Medicine, no. 168, 107804, 2024, doi.org/10.1016/j.compbiomed.2023.107804.
- M. F. Greenwald, B. A. Scruggs, J. M. Vislisel, and M. A. Greiner. ”Corneal imaging: an introduction.” Iowa City (Iowa): Department of Ophthalmology and Visual Sciences, University of Iowa Health Care 9, 2016.
- A., H. Alyaa, H. N. Ghaeb, and Z. M. Musa. ”Support vector machine for keratoconus detection by using topographic maps with the help of image processing techniques.” IOSR Journal of Pharmacy and Biological Sciences, vol. 12, no. 6, 2017, 50-58, doi.org/10.9790/3008-1206065058.
- M. C. Arbelaez, F. Versaci, G. Vestri, P. Barboni, and G. Savini. ”Use of a support vector machine for keratoconus and subclinical keratoconus detection by topographic and tomographic data.” Ophthalmology vol. 119, no. 11, 2012, 2231-2238, doi.org/10.1016/j.ophtha.2012.06.005.
- A. Lavric, P. Valentin, T. Hidenori, and S. Yousefi. ”Detecting keratoconus from corneal imaging data using machine learning.” IEEE Access vol. 8, 2020, 149113-149121, doi.org/10.1109/ACCESS.2020.3016060.
- K. Kamiya, Y. Ayatsuka, Y. Kato, F. Fujimura, M. Takahashi, N. Shoji, Y. Mori, and K. Miyata. “Keratoconus detection using deep learning of colour-coded maps with anterior segment optical coherence tomography: a diagnostic accuracy study.” BMJ open vol. 9, no. 9, 2019, doi.org/10.1136/bmjopen-2019-031313.
- L. M. Hartmann et al., “Keratoconus Progression Determined at the First Visit: A Deep Learning Approach With Fusion of Imaging and Numerical Clinical Data,” Translational Vision Science & Technology, vol. 13, no. 5, 2024, doi.org/10.1167/tvst.13.5.7
- A. Tillmann et al., “Acute corneal melt and perforation – a possible complication after riboflavin/UV-A crosslinking (CXL) in keratoconus.,”American journal of ophthalmology case reports, vol. 28, 101705, 2022, doi.org/10.1016/j.ajoc.2022.101705
- A. H. Al-Timemy, N. H. Ghaeb, Z. M. Mosa, and J. Escudero. “Deep transfer learning for improved detection of keratoconus using corneal topographic maps.” Cognitive Computation vol.14, no. 5, 2022, 1627-1642. doi.org/10.1007/s12559-021-09880-3
- K. Kazutaka, Y. Ayatsuka, Y. Kato, N. Shoji, Y. Mori, and K. Miyata. “Diagnosability of keratoconus using deep learning with Placido disk-based corneal topography. ” Frontiers in Medicine vol. 8, 2021, 724902, doi.org/10.3389/fmed.2021.724902
- I. Issarti, A. Consejo, M. Jiménez-García, S. Hershko, C. Koppen, and J. J. Rozema. “Computer aided diagnosis for suspect keratoconus detection. ” Computers in biology and medicine vol. 109, 2019, 33-42. doi.org/10.1016/j.compbiomed.2019.04.024
- B. R. Salem, and V. I. Solodovnikov. “Decision support system for an early-stage keratoconus diagnosis. ” In Journal of Physics: Conference Series, vol. 1419, no. 1, p. 012023. IOP Publishing, 2019, doi.org/10.1088/1742-6596/1419/1/012023
- X. Xu, T. Liang, J. Zhu, D. Zheng, and T. Sun. “Review of classical dimensionality reduction and sample selection methods for large-scale data processing,” Neurocomputing, vol. 328, 2019, 5-15, doi.org/10.1016/j.neucom.2018.02.100
- H. S. Hippert, C. E. Pedreira, and R. C. Souza. “Neural networks for short-term load forecasting: A review and evaluation,” IEEE Transactions on power systems vol. 16, no. 1, 2001, 44-55, doi.org/10.1109/59.910780
- E. Mocanu, P. H. Nguyen, M. Gibescu, and W. L. Kling. ”Deep learning for estimating building energy consumption. “Sustainable Energy, Grids and Networks 6, 2016, 91-99, doi.org/10.1016/j.segan.2016.02.005
- M. R. Arahal, A. Cepeda, and E. F. Camacho. ”Input variable selection for forecasting models. “ IFAC Proceedings, Vol. 35, no. 1, 2002, 463-468, doi.org/10.3182/20020721-6-ES-1901.00730
- Fan, Cheng, Fu Xiao, and Yang Zhao. “A short-term building cooling load prediction method using deep learning algorithms. ”Applied energy vol. 195, 2017, 222-233, doi.org/10.1016/j.apenergy.2017.03.064
- S. Hochreiter, and J. Schmidhuber. “Long short-term memory. ” Neural computation vol. 9, no. 8, 1997, 1735-1780, doi.org/10.1162/neco.1997.9.8.1735