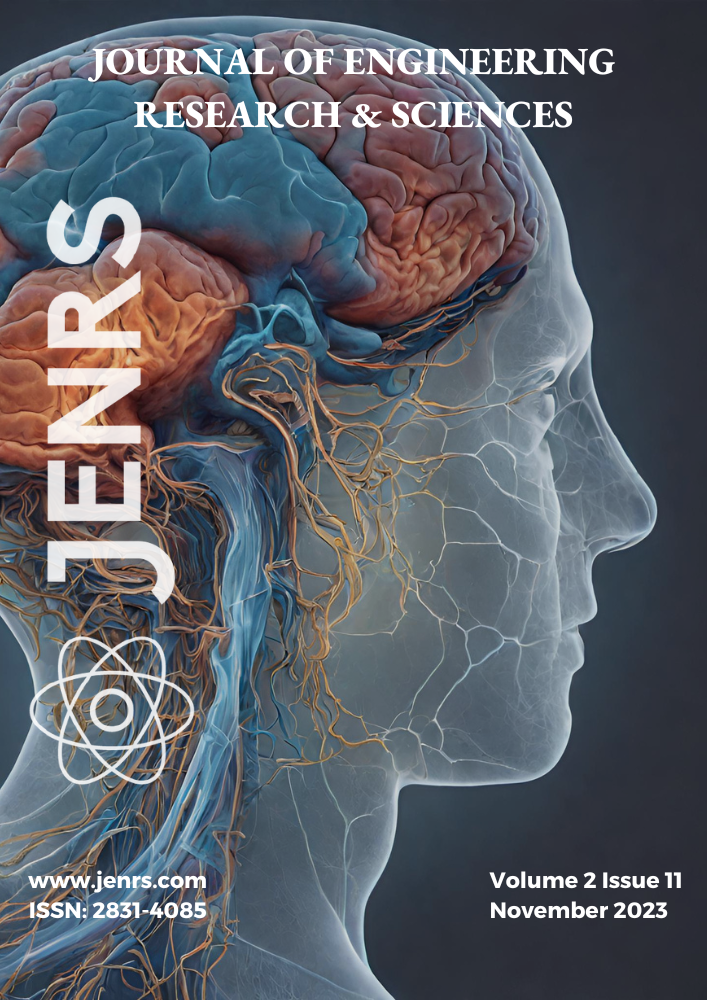
Volume 2, Issue 11
Download Complete Issue
This issue explores recent progress in computational neuroscience and machine learning, with a focus on Liquid State Machines (LSMs). These brain-inspired neural networks can handle changing inputs over time. The featured research paper looks at how the way LSMs represent information affects their performance. It suggests a new model that uses spike timing patterns instead of just counting spikes. This approach is closer to how real brains work and could make LSMs better at solving classification problems. The study shows that paying attention to when spikes happen, not just how many there are, can capture important information that older methods missed.
Front Cover
Publication Month: November 2023, Page(s): i – iÂ
Editorial Board
Publication Month: November 2023, Page(s): ii – ii
Editorial
Publication Month: November 2023, Page(s): iii – iii
Table of Contents
Publication Month: November 2023, Page(s): iv – iv
Neural Synchrony-Based State Representation in Liquid State Machines, an Exploratory Study
Nicolas Pajot, Mounir Boukadoum
J. Engg. Res. & Sci. 2(11), 1-14 (2023);
Solving classification problems by Liquid State Machines (LSM) usually ignores the influence of the liquid state representation on performance, leaving the role to the reader circuit. In most studies, the decoding of the internally generated neural states is performed on spike rate-based vector representations. This approach occults the interspike timing, a central aspect of biological neural coding, with potentially detrimental consequences on the LSM performance. In this work, we propose a model of liquid state representation that builds the feature vectors from temporal information extracted from the spike trains, hence using spike synchrony instead of rate. Using pairs of Poisson-distributed spike trains in noisy conditions, we show that such model outperforms a rate-only model in distinguishing two spike trains regardless of the sampling frequency of the liquid states or the noise level. In the same vein, we suggest a synchrony-based measure of the separation property (SP), a core feature of LSMs regarding classification performance, for a more robust and biologically plausible interpretation.