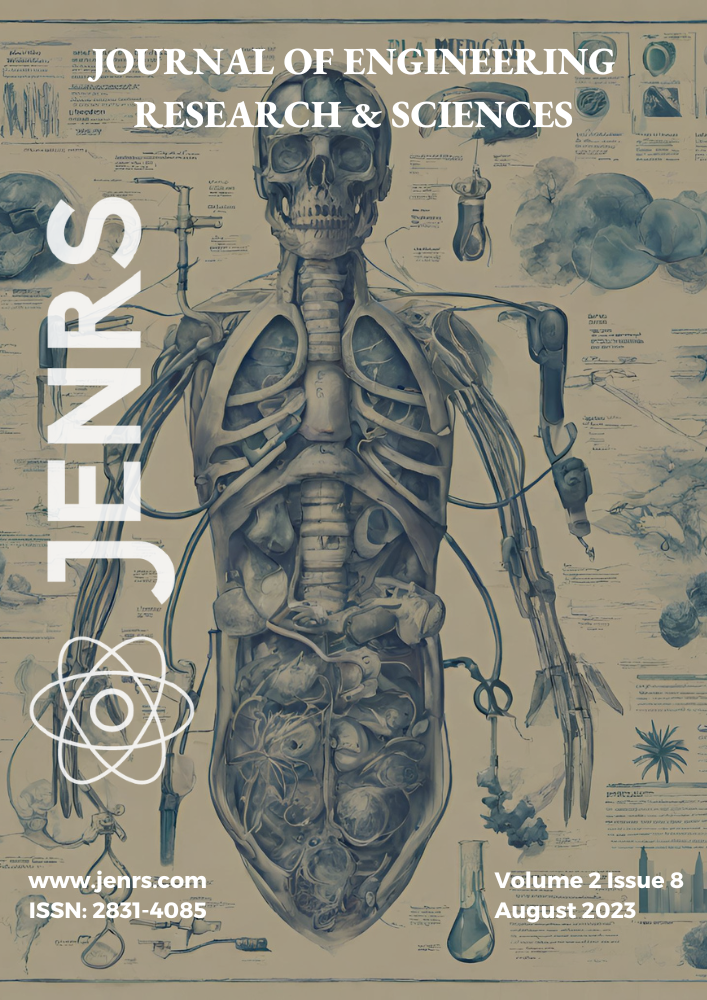
Front Cover
Publication Month: January 2022, Page(s): A1 – A1
Editorial Board
Publication Month: January 2022, Page(s): B1 – B1
Editorial
Publication Month: January 2022, Page(s): C1 – C1
Table of Contents
Publication Month: January 2022, Page(s): D1 – D1
Imputation and Hyperparameter Optimization in Cancer Diagnosis
Yi Liu, Wendy Wang, Haibo Wang
J. Engg. Res. & Sci. 2(8), 1-18 (2023);
Cancer is one of the leading causes for death worldwide. Accurate and timely detection of cancer can save lives. As more machine learning algorithms and approaches have been applied in cancer diagnosis, there has been a need to analyze their performance. This study has compared the detection accuracy and speed of nineteen machine learning algorithms using a cervical cancer dataset. To make the approach general enough to detect various types of cancers, this study has intentionally excluded feature selection, a feature commonly applied in most studies for a specific dataset or a certain type of cancer. In addition, imputation and hyperparameter optimization have been employed to improve the algorithms’ performance. The results suggest that when both imputation and hyperparameter optimization are applied, the algorithms tend to perform better than when either of them is employed individually or when both are absent. The majority of the algorithms have shown improved accuracy in diagnosis, although with the trade-off of increased execution time. The findings from this study demonstrate the potential of machine learning in cancer diagnosis, especially the possibility of developing versatile systems that are able to detect various types of cancers with satisfactory performance.